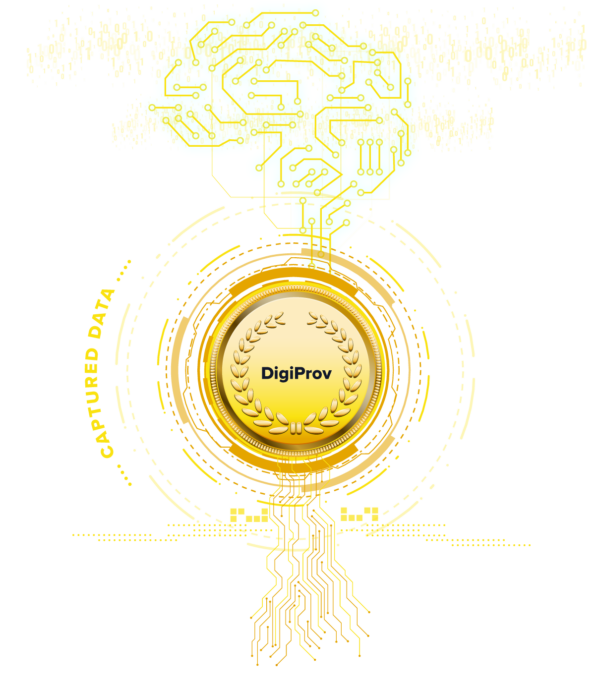
The pressing weight of unknown data history
Data provenance is information held as metadata that details origin and change history which – in turn – helps to establish confidence in and validity of that data. Provenance is an acute issue in a number of industries where accurate, trusted data is vital: for providing credible change history to guard against litigation; ensuring efficiency and the ability to ‘roll back’; and for delivering successful business outcomes. Provenance is currently an acute issue in scientific databases where it is central to the validation of data, in financial businesses for confirming pedigree of information, in deriving correct insights from data generated by Internet of Things (IoT) devices, and in asset management data.
Although many individual data handling systems are delivered with provenance capability as standard, the provenance only exists within that limited system. Obtaining provenance across systems and tools as individuals use it for analysis and insights can be a labour intensive, costly undertaking. With a huge volume of new data being created without such tracking, a solution for assigning post facto provenance is long overdue.
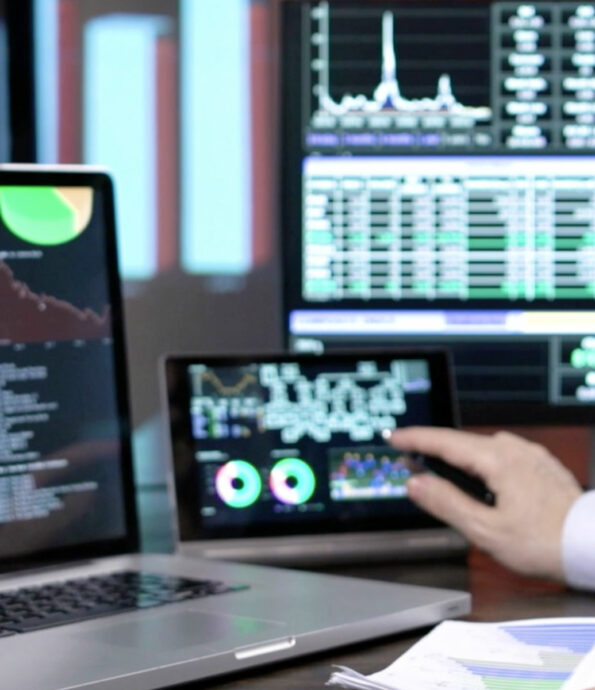
Automatic, intelligent derivation of meaningful provenance
The DigiProv solution has been designed to intelligently infer an accurate provenance retrospectively by using AI to trace and complete the history of data. This solution tackles two key elements: evaluating where provenance must be captured (and where it is not required); and defining shortcuts that can be taken to infer meaningful provenance where none is available.
The DigiProv solution can accurately derive the evolution of data by following typical, assumed paths, and learning as it maps.
This solution aims to significantly reduce the cost and effort required to create essential provenance and could be offered as a specialist consultant-led solution, or further developed in-house within a particular vertical.
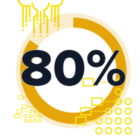
80% of asset managers admit that they are still only in the early stages of understanding data lineage, an essential part of their ability to operate efficiently.
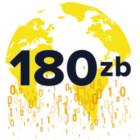
180zb data growth by 2025. Global data creation is growing exponentially with creation rates p/annum to reach 180 zettabytes by 2025, almost 119zb more than in 2020.
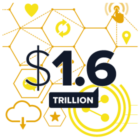
$1.6trillion global IoT market by 2025. The market for IoT solutions is expected to grow to $1.6 trillion by 2025 (from $212 billion in 2019). The IoT is expected to be one of the biggest generators of world data.
From the Inventor and Project Lead
This invention was born from research – following years of consulting work with the US Federal Government – where I saw the same problem of missing or incomplete data provenance over and over again. I am thrilled we have been able to create a practical and effective solution that can be applied to a diverse range of systems and has the potential to return vast cost and time saving benefits.

Progress
Initial development complete
The system has been automated – managed by a bespoke, open-source software – and a user interface has been developed to allow users to set up measurement parameters to run tests, and read and interpret results.
Ready to move into software engineering phase
With design and initial development complete, the DigiProv team are now looking for investment into resources to continue the software engineering phase.
Exploring the potential
The potential for the innovation is ready to be explored in earnest with application of the solution in specific industry(s). Initially this could take the form of a consultant-led engagement or the solution could be developed in-house.
Meet the DigiProv Team
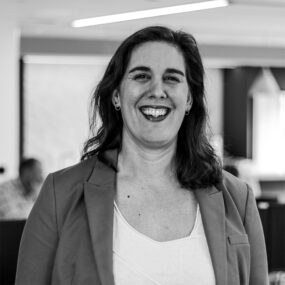
Age Chapman is Professor of Computer Science and Head of the Digital Health group, University of Southampton. Prior to coming to the UK, she worked on a variety of projects with the US Federal government, including the Office of the National Coordinators (ONC) report on provenance in electronic health systems. She has advised the US Food and Drug Agency (FDA), the National Geospatial-Intelligence Agency (NGA), and the Department of Homeland Security (DHS) on data management. Her research focuses on database systems, and she chairs the steering committee of the Theory and Practice of Provenance (TaPP) and ProvenanceWeek. She is also the recipient of the 2016 ACM SIGMOD Test of Time Award for her work on provenance.
More information
The team is looking for investors, industry partners or data specialists who recognise the challenge of data provenance and the cost saving, efficiency-driving opportunity that this unique solution could bring to a wide number of industries.
The market potentials are significant, with the ability to accurately derive provenance from data delivering the opportunity for asset managers, financial analysts, healthcare, defence, government departments and scientific research teams to accurately trace the history of data in an efficient and cost-effective way.
In the finance and commercial sectors: Gartner found that the average cost of poor data quality on businesses amounts to anywhere between $9.7 million and $14.2 million annually. At the macro level, bad data is estimated to cost the US more than $3 trillion per year. In other words, bad data and poor provenance is bad for business. [gartner report]
In the science sector: A PWC report for the EU found that the annual cost of not having FAIR research data costs the European economy at least €10.2bn every year. In addition, they listed consequences from not having FAIR including an impact on research quality, economic turnover, or machine readability of research data. PWC estimated that these unquantified elements could account for another €16bn annually. [pwc report for the eu]
Get in touch
"*" indicates required fields