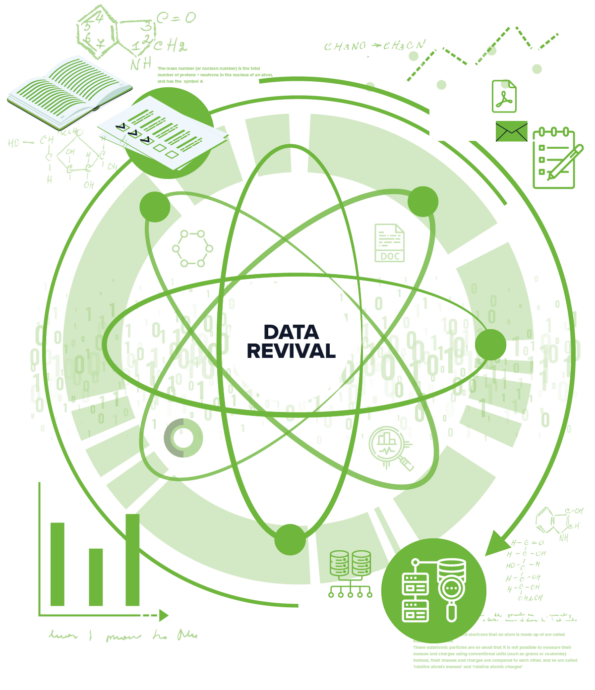
The untapped potential of scientific data
There is a vast amount of historical, unstructured data lying under-utilised across industry sectors, and more continues to be generated every day. A particular issue in the scientific community, a vast array of digital data – such as pdfs, imagery, and databases – is unsearchable, while vital historic data is largely held in a physical format, with typed reports sitting in files alongside structure diagrams and annotated data charts.
Accessing this data is notoriously complex and labour-intensive, costing businesses millions in man hours. Both knowledge leakage – where archival knowledge is held with experienced individuals – and speed to discovery is also impacted in pharmaceutical sciences and across the wider chemical sciences industry representing a large scale missed opportunity cost.
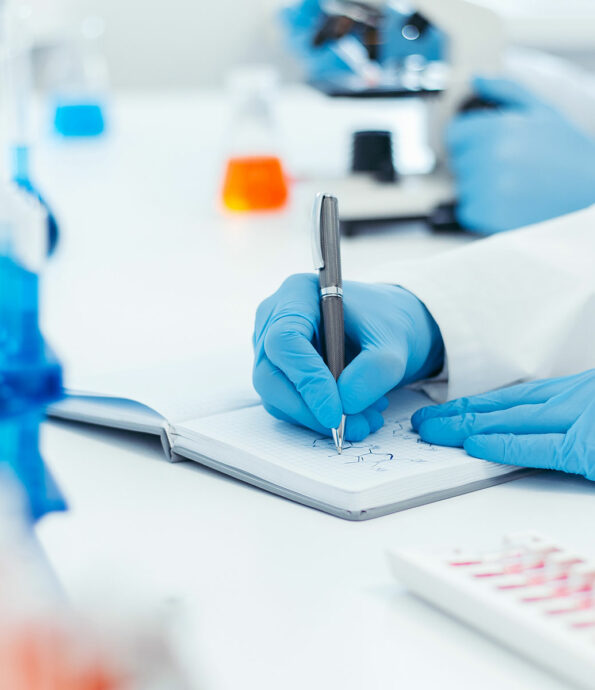
An intelligent system to fast track discovery and reduce cost
The Data Revival system can quickly extract information from any document format with a high degree of accuracy, creating meaningful, accurate links between information types – such as charts and their labels, or chemical structures and their written formula.
Unlike other data extraction software, Data Revival uses built-in, chemistry-based contextualization to recognise sector-specific terminology including trade, official and esoteric names. Using deep-learning AI neural networks, Data Revival continually extends its capabilities as it parses information from both structured and unstructured data, essentially extending its knowledge over time.
Well-proven in a chemical sciences context, Data Revival already demonstrates high potential for other sectors – such as law and engineering – with further development of the semantic search capabilities.
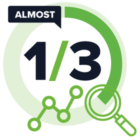
Almost one third of employees’ time is spent searching for data. The IDC found that – across all industries – about 2.5 hours per day, or roughly 30% of the workday, is spent searching for information. In the oil and gas industry this is estimated to be far worse, at almost 80%.
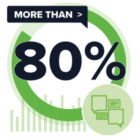
More than 80% of business data is unstructured. All industries suffer from the issue of unstructured data, with 80% of all business-held information lying in sources such as emails, pdfs, and hard copy. There is a widespread need to invest in advanced analytics – using AI and neural learning – to unlock the potential of this data.
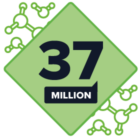
37 million unique molecules held in the PubChem database. The scale of data in the chemical industry, and its relative value, is vast. The ability to mine that data in a fast, efficient, and meaningful way – such as searching for drug-like molecules based on their structural features, complexity, or application – opens huge opportunities across the sector.
From Data Revival’s Co-Founder
The exciting thing about Data Revival isn’t just its unique ability to draw meaning out of unstructured data, it’s the almost boundless application potential. We’ve already run successful pilots at a multinational chemical company and academic institutions and have seen interest from the legal and healthcare sectors. With the right backing I believe Data Revival has the potential to become the Google for unstructured data.

Progress
Successful Trial with European Chemical Company
Data Revival have run a trial with a multi-national €2.1 billion European Chemical Company across a range of document types. The business is now using the system operationally and looking to expand to a wider remit.
Market Validation Shows Widespread Interest
The ICURe 3-month program focused on market validation from 135 companies in 20 different sectors, across 9 countries. Data Revival saw notable interest across several sectors outside the chemical industry – including a large law firm and a healthcare provider.
Pilot Projects Running Across UoS
Data Revival are running a pilot with Southampton University digitising and extracting information from the Chemistry department COSHH forms and lab books. This will support potential audits, as well as saving money and space for storage of lab books. Similar pilots across other departments are being planned.
Meet the Data Revival team
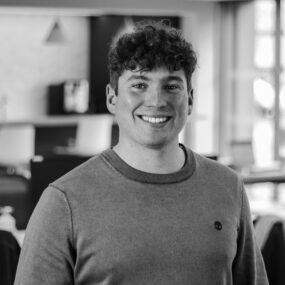
Samuel Munday is a Senior Research Assistant at the University of Southampton and led the development and implementation of a machine learning platform for the polymeric materials sector, aiding them in bringing new products to market faster. He developed and worked in the team to deliver a Python programming course for undergraduate Chemists as well as assessing the ethical implications of implementing AI and data sharing across the food supply chain.
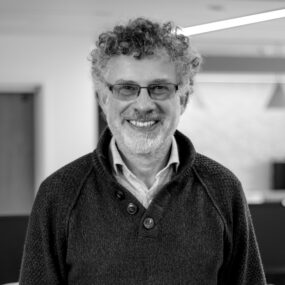
Professor Jeremy G Frey is Professor of Physical Chemistry within Chemistry at the University of Southampton, and a Turing Fellow. He investigates how e-Science infrastructure supports scientific research, making use of AI and Machine Learning, with an emphasis on the way digital infrastructure can enhance the intelligent creation, dissemination, and analysis of scientific data.
More information
We are looking for investment, partnership, or funding to further expand the capabilities of the Data Revival system to the point where it is market ready.
Investment will allow us to bring in development resources to work on the front-end GUI, and back end support to extend the management and query systems. We will continue to work to refine the computational relevance in the field of chemical sciences and – long term – would want to be in a position to engage specialists to support us in extending the application in the context of other sectors.
While working in a business focused on polymers, we found that we needed to access and use archaic document formats to carry out research-centric objectives.
The business wanted to create a machine learning system to predict the properties of polymers that might be suitable for development and production. This system would drive the direction of experimentation in the lab and allow the business to explore many, many more chemical formulae in far less time.
But you can’t build a prediction model without a valuable data set. And the more significant data set, the more accurate the predictions.
The vast array of data available was largely sitting in filing cabinets. The numerical data needed to be fed manually into spreadsheets. We realised that, in doing so, we were losing a valuable part of the data – its context. Take for example two polymers that have the same molecular structure. Only the contextual information tells us that processing these at 20 degrees or 200 degrees have resulted in polymers with entirely different properties at the end point.
To tackle the problem we set about building the origins of what is now Data Revival. The programme was able to find patterns and make predictions that would take an individual many months, or even years, to calculate. As a result, the business was able to take three new polymers to the lab, one of which has been taken forward for potential production, in a fraction of the time it would have taken to do manually.
In our trial with Allnex the data included 80% chemistry material augmented by 20% related business information, including emails, receipts, etc, that added to the story told by the chemical reports. Without Data Revival capturing and recording the relational links between these information types a substantial portion of the value of that data to any R&D project would have been lost.
We are currently running a Data Revival IAA project with Bristol University to pull in open source PhD theses and make the content available through Data Revival. To date, these valuable resources are not being used effectively as they are hard to search in a meaningful way. A pilot study done previously manually curated compounds from a selection of open source thesis and found many compounds were new to the wider community with a significant proportion being potentially useful (https://pubs.rsc.org/en/content/articlehtml/2016/sc/c6sc00264a).
Data Revival are expanding on this manual pilot with an automatic curation and the provision of digital search.
Get in touch
"*" indicates required fields